Machine Learning - Standard Deviation
What is Standard Deviation?
Standard deviation is a number that describes how spread out the values are.
A low standard deviation means that most of the numbers are close to the mean (average) value.
A high standard deviation means that the values are spread out over a wider range.
Example: This time we have registered the speed of 7 cars:
speed = [86,87,88,86,87,85,86]
The standard deviation is:
0.9
Meaning that most of the values are within the range of 0.9 from the mean value, which is 86.4.
Let us do the same with a selection of numbers with a wider range:
speed = [32,111,138,28,59,77,97]
The standard deviation is:
37.85
Meaning that most of the values are within the range of 37.85 from the mean value, which is 77.4.
As you can see, a higher standard deviation indicates that the values are spread out over a wider range.
The NumPy module has a method to calculate the standard deviation:
Example
Use the NumPy std()
method to find the
standard deviation:
import numpy
speed = [86,87,88,86,87,85,86]
x = numpy.std(speed)
print(x)
Try it Yourself »
Learn to Filter Data in Python Like a Data Analyst
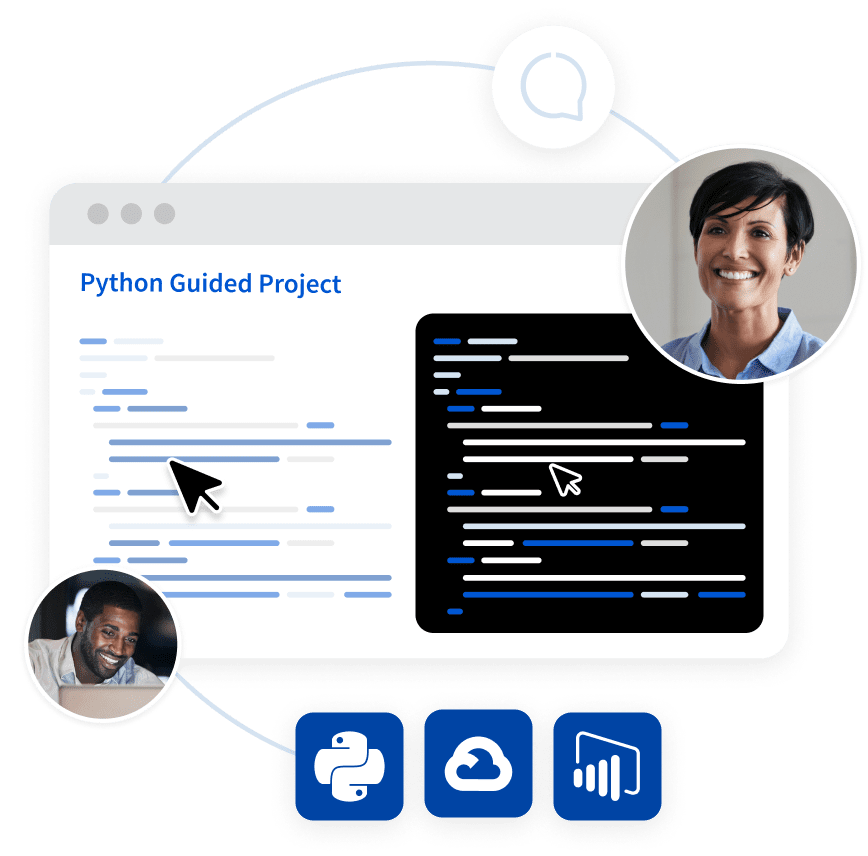
Try a hands-on training sessions with step-by-step guidance from an expert. Try the guided project made in collaboration with Coursera now!
Get startedVariance
Variance is another number that indicates how spread out the values are.
In fact, if you take the square root of the variance, you get the standard deviation!
Or the other way around, if you multiply the standard deviation by itself, you get the variance!
To calculate the variance you have to do as follows:
1. Find the mean:
(32+111+138+28+59+77+97) / 7 = 77.4
2. For each value: find the difference from the mean:
32 - 77.4 = -45.4
111 - 77.4 = 33.6
138
- 77.4 = 60.6
28 - 77.4 = -49.4
59 - 77.4 = -18.4
77
- 77.4 = - 0.4
97 - 77.4 = 19.6
3. For each difference: find the square value:
(-45.4)2 = 2061.16
(33.6)2 = 1128.96
(60.6)2 = 3672.36
(-49.4)2 = 2440.36
(-18.4)2 = 338.56
(- 0.4)2 = 0.16
(19.6)2 = 384.16
4. The variance is the average number of these squared differences:
(2061.16+1128.96+3672.36+2440.36+338.56+0.16+384.16)
/ 7 = 1432.2
Luckily, NumPy has a method to calculate the variance:
Example
Use the NumPy var()
method to find the variance:
import numpy
speed = [32,111,138,28,59,77,97]
x = numpy.var(speed)
print(x)
Try it Yourself »
Standard Deviation
As we have learned, the formula to find the standard deviation is the square root of the variance:
√1432.25 = 37.85
Or, as in the example from before, use the NumPy to calculate the standard deviation:
Example
Use the NumPy std()
method to find the standard deviation:
import numpy
speed = [32,111,138,28,59,77,97]
x = numpy.std(speed)
print(x)
Try it Yourself »
Symbols
Standard Deviation is often represented by the symbol Sigma: σ
Variance is often represented by the symbol Sigma Squared: σ2
Chapter Summary
The Standard Deviation and Variance are terms that are often used in Machine Learning, so it is important to understand how to get them, and the concept behind them.